Sensitive Data Classification Challenges Security Teams Face
Ensuring the security of your data involves more than just pinpointing its location. It's a multifaceted process in which knowing where your data resides is just the initial step. Beyond that, accurate classification plays a pivotal role. Picture it like assembling a puzzle – having all the pieces and knowing their locations is essential, but the real mastery comes from classifying them (knowing which belong to the edge, which make up the sky in the picture, and so on…), seamlessly creating the complete picture for your proper data security and privacy programs.
Just last year, the global average cost of a data breach surged to USD 4.45 million, a 15% increase over the previous three years. This highlights the critical need to automatically discover and accurately classify personal and unique identifiers, which can transform into sensitive information when combined with other data points.
This unique capability is what sets Sentra’s approach apart— enabling the detection and proper classification of data that many solutions overlook or mis-classify.
What Is Data Classification and Why Is It Important?
Data classification is the process of organizing and labeling data based on its sensitivity and importance. This involves assigning categories like "confidential," "internal," or "public" to different types of data. It’s further helpful to understand the ‘context’ of data - it’s purpose - such as legal agreements, health information, financial record, source code/IP, etc. With data context you can more precisely understand the data’s sensitivity and accurately classify it (to apply proper policies and related violation alerting, eliminating false positives as well).
Here's why data classification is crucial in the cloud:
- Enhanced Security: By understanding the sensitivity of your data, you can implement appropriate security measures. Highly confidential data might require encryption or stricter access controls compared to publicly accessible information.
- Improved Compliance: Many data privacy regulations require organizations to classify personally identifying data to ensure its proper handling and protection. Classification helps you comply with regulations like GDPR or HIPAA.
- Reduced Risk of Breaches: Data breaches often stem from targeted attacks on specific types of information. Classification helps identify your most valuable data assets, so you can apply proper controls and minimize the impact of a potential breach.
- Efficient Management: Knowing what data you have and where it resides allows for better organization and management within the cloud environment. This can streamline processes and optimize storage costs.
Data classification acts as a foundation for effective data security. It helps prioritize your security efforts, ensures compliance, and ultimately protects your valuable data. Securing your data and mitigating privacy risks begins with a data classification solution that prioritizes privacy and security. Addressing various challenges necessitates a deeper understanding of the data, as many issues require additional context.
The end goal is automating processes and making findings actionable - which requires granular, detailed context regarding the data’s usage and purpose, to create confidence in the classification result.
In this article, we will define toxic combinations and explore specific capabilities required from a data classification solution to tackle related data security, compliance, and privacy challenges effectively.
Data Classification Challenges
Challenge 1: Unstructured Data Classification
Unstructured data is information that lacks a predefined format or organization, making it challenging to analyze and extract insights, yet it holds significant value for organizations seeking to leverage diverse data sources for informed decision-making. Examples of unstructured data include customer support chat logs, educational videos, and product photos. Detecting data classes within unstructured data with high accuracy poses a significant challenge, particularly when relying solely on simplistic methods like regular expressions and pattern matching. Unstructured data, by its very nature, lacks a predefined and organized format, making it challenging for conventional classification approaches. Legacy solutions often grapple with the difficulty of accurately discerning data classes, leading to an abundance of false positives and noise.
This highlights the need for more advanced and nuanced techniques in unstructured data classification to enhance accuracy and reduce its inherent complexities. Addressing this challenge requires leveraging sophisticated algorithms and machine learning models capable of understanding the intricate patterns and relationships within unstructured data, thereby improving the precision of data class detection.
In the search for accurate data classification within unstructured data, incorporating technologies that harness machine learning and artificial intelligence is critical. These advanced technologies possess the capability to comprehend the intricacies of context and natural language, thereby significantly enhancing the accuracy of sensitive information identification and classification.
For example, detecting a residential address is challenging because it can appear in multiple shapes and forms, and even a phone number or a GPS coordinate can be easily confused with other numbers without fully understanding the context. However, LLMs can use text-based classification techniques (NLP, keyword matching, etc.) to accurately classify this type of unstructured data. Furthermore, understanding the context surrounding each data asset, whether it be a table or a file, becomes paramount. Whether it pertains to a legal agreement, employee contract, e-commerce transaction, intellectual property, or tax documents, discerning the context aids in determining the nature of the data and guides the implementation of appropriate security measures. This approach not only refines the accuracy of data class detection but also ensures that the sensitivity of the unstructured data is appropriately acknowledged and safeguarded in line with its contextual significance.
Optimal solutions employ machine learning and AI technology that really understand the context and natural language in order to classify and identify sensitive information accurately. Advancements in technologies have expanded beyond text-based classification to image-based classification and audio/speech-based classification, enabling companies and individuals to efficiently and accurately classify sensitive data at scale.
Challenge 2: Customer Data vs Employee Data
Employee data and customer data are the most common data categories stored by companies in the cloud. Identifying customer and employee data is extremely important. For instance, customer data that also contains Personal Identifiable Information (PII) must be stored in compliant production environments and must not travel to lower environments such as data analytics or development.
- What is customer data?
Customer data is all the data that we store and collect from our customers and users.
- B2C - Customer data in B2C companies, includes a lot of PII about their end users, all the information they transact with our service.
- B2B - Customer data in B2B companies includes all the information of the organization itself, such as financial information, technological information, etc., depending on the organization.
This could be very sensitive information about each organization that must remain confidential or otherwise can lead to data breaches, intellectual property theft, reputation damage, etc.
- What is employee data?
Employee data includes all the information and knowledge that the employees themselves produce and consume. This could include many types of different information, depending on what team it comes from.
For instance:
-Tech and intellectual property, source code from the engineering team.
-HR information, from the HR team.
-Legal information from the legal team, source code, and many more.
It is crucial to properly classify employee and customer data, and which data falls under which category, as they must be secured differently. A good data classification solution needs to understand and differentiate the different types of data. Access to customer data should be restricted, while access to employee data depends on the organizational structure of the user’s department. This is important to enforce in every organization.
Challenge 3: Understanding Toxic Combinations
What Is a Toxic Combination?
A toxic combination occurs when seemingly innocuous data classes are combined to increase the sensitivity of the information. On their own, these pieces of information are harmless, but when put together, they become “toxic”.
The focus here extends beyond individual data pieces; it's about understanding the heightened sensitivity that emerges when these pieces come together. In essence, securing your data is not just about individual elements but understanding how these combinations create new vulnerabilities.
We can divide data findings into three main categories:
- Personal Identifiers: Piece of information that can identify a single person - for example, an email address or social security number (SSN), belongs only to one person.
- Personal Quasi Identifiers: A quasi identifier is a piece of information that by itself is not enough to identify just one person. For example, a zip code, address, an age, etc. Let’s say Bob - there are many Bobs in the world, but if we also have Bob’s address - there is most likely just one Bob living in this address.
- Sensitive Information: Each piece of information that should remain sensitive/private. Such as medical diseases, history, prescriptions, lab results, etc. automotive industry - GPS location. Sensitive data on its own is not sensitive, but the combination of identifiers with sensitive information is very sensitive.
.webp)
Finding personal identifiers by themselves, such as an email address, does not necessarily mean that the data is highly sensitive. Same with sensitive data such as medical info or financial transactions, that may not be sensitive if they can not be associated with individuals or other identifiable entities.
However, the combination of these different information types, such as personal identifiers and sensitive data together, does mean that the data requires multiple data security and protection controls and therefore it’s crucial that the classification solution will understand that.
Detecting ‘Toxic Data Combinations’ With a Composite Class Identifier
Sentra has introduced a new ‘Composite’ data class identifier to allow customers to easily build bespoke ‘toxic combinations’ classifiers they wish for Sentra to deploy to identify within their data sets.
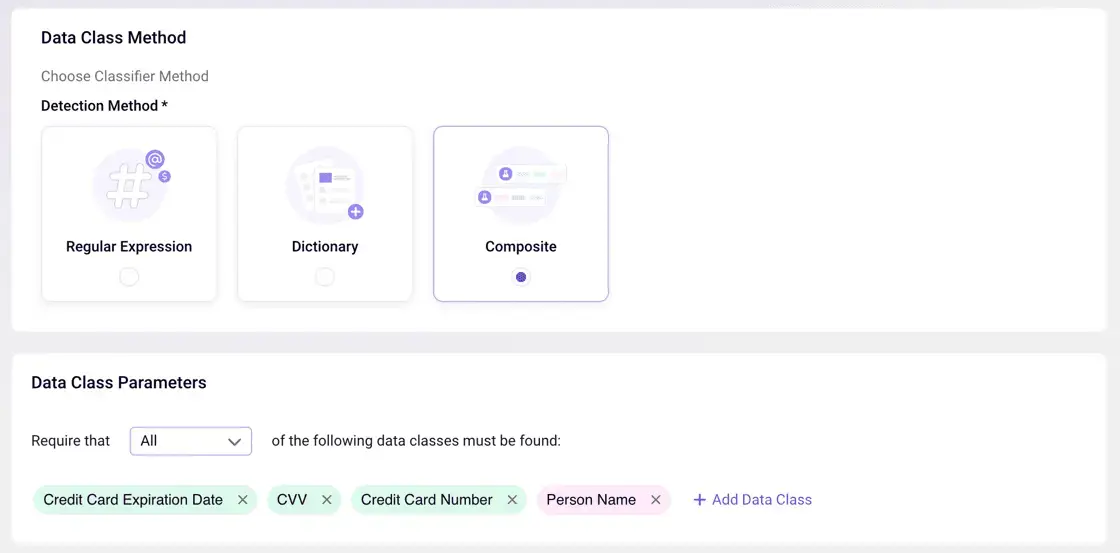
Importance of Finding Toxic Combinations
This capability is critical because having sensitive information about individuals can harm the business reputation, or cause them fines, privacy violations, and more. Under certain data privacy and protection requirements, this is even more crucial to discover and be aware of. For example, HIPAA requires protection of patient healthcare data. So, if an individual’s email is combined with his address, and his medical history (which is now associated with his email and address), this combination of information becomes sensitive data.
Challenge 4: Detecting Uncommon Personal Identifiers for Privacy Regulations
There are many different compliance regulations, such as Privacy and Data Protection Acts, which require organizations to secure and protect all personally identifiable information. With sensitive cloud data constantly in flux, there are many unknown data risks arising. This is due to a lack of visibility and an inaccurate data classification solution.Classification solutions must be able to detect uncommon or proprietary personal identifiers. For example, a product serial number that belongs to a specific individual, U.S. Vehicle Identification Number (VIN) might belong to a specific car owner, or GPS location that indicates an individual home address can be used to identify this person in other data sets.
These examples highlight the diverse nature of identifiable information. This diversity requires classification solutions to be versatile and capable of recognizing a wide range of personal identifiers beyond the typical ones.
Organizations are urged to implement classification solutions that both comply with general privacy and data protection regulations and also possess the sophistication to identify and protect against a broad spectrum of personal identifiers, including those that are unconventional or proprietary in nature. This ensures a comprehensive approach to safeguarding sensitive information in accordance with legal and privacy requirements.
Challenge 5: Adhering to Data Localization Requirements
Data Localization refers to the practice of storing and processing data within a specific geographic region or jurisdiction. It involves restricting the movement and access to data based on geographic boundaries, and can be motivated by a variety of factors, such as regulatory requirements, data privacy concerns, and national security considerations.
In adherence to the Data Localization requirements, it becomes imperative for classification solutions to understand the specific jurisdictions associated with each of the data subjects that are found in Personal Identifiable Information (PII) they belong to.For example, if we find a document with PII, we need to know if this PII belongs to Indian residents, California residents or German citizens, to name a few. This will then dictate, for example, in which geography this data must be stored and allow the solution to indicate any violations of data privacy and data protection frameworks, such as GDPR, CCPA or DPDPA.
Below is an example of Sentra’s Monthly Data Security Report: GDPR
.webp)
.png)
Why Data Localization Is Critical
- Adhering to local laws and regulations: Ensure data storage and processing within specific jurisdictions is a crucial aspect for organizations. For instance, certain countries mandate the storage and processing of specific data types, such as personal or financial data, within their borders, compelling organizations to meet these requirements and avoid potential fines or penalties.
- Protecting data privacy and security: By storing and processing data within a specific jurisdiction, organizations can have more control over who has access to the data, and can take steps to protect it from unauthorized access or breaches. This approach allows organizations to exert greater control over data access, enabling them to implement measures that safeguard it from unauthorized access or potential breaches.
- Supporting national security and sovereignty: Some countries may want to store and process data within their borders. This decision is driven by the desire to have more control over their own data and protect their citizens' information from foreign governments or entities, emphasizing the role of data localization in supporting these strategic objectives.
Conclusion: Sentra’s Data Classification Solution
Sentra provides the granular classification capabilities to discern and accurately classify the formerly difficult to classify data types just mentioned. Through a variety of analysis methods, we address those data types and obscure combinations that are crucial to effective data security. These combinations too often lead to false positives and disappointment in traditional classification systems.
In review, Sentra’s data classification solution accurately:
- Classifies Unstructured data by applying advanced AI/ML analysis techniques
- Discerns Employee from Customer data by analyzing rich business context
- Identifies Toxic Combinations of sensitive data via advanced data correlation techniques
- Detects Uncommon Personal Identifiers to comply with stringent privacy regulations
- Understands PII Jurisdiction to properly map to applicable sovereignty requirements
To learn more, visit Sentra’s data classification use case page or schedule a demo with one of our experts.
<blogcta-big>