Use Redshift Data Scrambling for Additional Data Protection
According to IBM, a data breach in the United States cost companies an average of 9.44 million dollars in 2022. It is now more important than ever for organizations to place high importance on protecting confidential information. Data scrambling, which can add an extra layer of security to data, is one approach to accomplish this.
In this post, we'll analyze the value of data protection, look at the potential financial consequences of data breaches, and talk about how Redshift Data Scrambling may help protect private information.
The Importance of Data Protection
Data protection is essential to safeguard sensitive data from unauthorized access. Identity theft, financial fraud,and other serious consequences are all possible as a result of a data breach. Data protection is also crucial for compliance reasons. Sensitive data must be protected by law in several sectors, including government, banking, and healthcare. Heavy fines, legal problems, and business loss may result from failure to abide by these regulations.
Hackers employ many techniques, including phishing, malware, insider threats, and hacking, to get access to confidential information. For example, a phishing assault may lead to the theft of login information, and malware may infect a system, opening the door for additional attacks and data theft.
So how to protect yourself against these attacks and minimize your data attack surface?
What is Redshift Data Masking?
Redshift data masking is a technique used to protect sensitive data in Amazon Redshift; a cloud-based data warehousing and analytics service. Redshift data masking involves replacing sensitive data with fictitious, realistic values to protect it from unauthorized access or exposure. It is possible to enhance data security by utilizing Redshift data masking in conjunction with other security measures, such as access control and encryption, in order to create a comprehensive data protection plan.
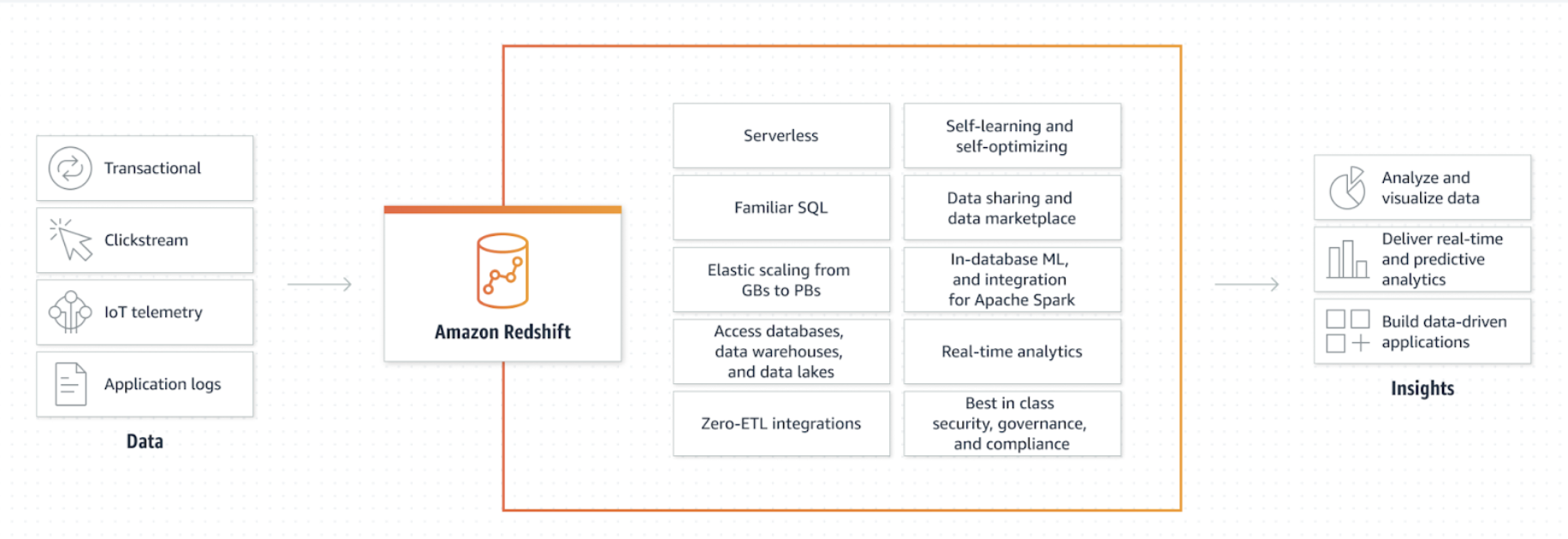
What is Redshift Data Scrambling?
Redshift data scrambling protects confidential information in a Redshift database by altering original data values using algorithms or formulas, creating unrecognizable data sets. This method is beneficial when sharing sensitive data with third parties or using it for testing, development, or analysis, ensuring privacy and security while enhancing usability.
The technique is highly customizable, allowing organizations to select the desired level of protection while maintaining data usability. Redshift data scrambling is cost-effective, requiring no additional hardware or software investments, providing an attractive, low-cost solution for organizations aiming to improve cloud data security.
Data Masking vs. Data Scrambling
Data masking involves replacing sensitive data with a fictitious but realistic value. However, data scrambling, on the other hand, involves changing the original data values using an algorithm or a formula to generate a new set of values.
In some cases, data scrambling can be used as part of data masking techniques. For instance, sensitive data such as credit card numbers can be scrambled before being masked to enhance data protection further.
Setting up Redshift Data Scrambling
Having gained an understanding of Redshift and data scrambling, we can now proceed to learn how to set it up for implementation. Enabling data scrambling in Redshift requires several steps.
To achieve data scrambling in Redshift, SQL queries are utilized to invoke built-in or user-defined functions. These functions utilize a blend of cryptographic techniques and randomization to scramble the data.
The following steps are explained using an example code just for a better understanding of how to set it up:
Step 1: Create a new Redshift cluster
Create a new Redshift cluster or use an existing cluster if available.

Step 2: Define a scrambling key
Define a scrambling key that will be used to scramble the sensitive data.
In this code snippet, we are defining a scrambling key by setting a session-level parameter named <inlineCode>my_scrambling_key<inlineCode> to the value <inlineCode>MyScramblingKey<inlineCode>. This key will be used by the user-defined function to scramble the sensitive data.
Step 3: Create a user-defined function (UDF)
Create a user-defined function in Redshift that will be used to scramble the sensitive data.
Here, we are creating a UDF named <inlineCode>scramble<inlineCode> that takes a string input and returns the scrambled output. The function is defined as <inlineCode>STABLE<inlineCode>, which means that it will always return the same result for the same input, which is important for data scrambling. You will need to input your own scrambling logic.
Step 4: Apply the UDF to sensitive columns
Apply the UDF to the sensitive columns in the database that need to be scrambled.
For example, applying the <inlineCode>scramble<inlineCode> UDF to a column saying, <inlineCode>ssn<inlineCode> in a table named <inlineCode>employee<inlineCode>. The <inlineCode>UPDATE<inlineCode> statement calls the <inlineCode>scramble<inlineCode> UDF and updates the values in the <inlineCode>ssn<inlineCode> column with the scrambled values.
Step 5: Test and validate the scrambled data
Test and validate the scrambled data to ensure that it is unreadable and unusable by unauthorized parties.
In this snippet, we are running a <inlineCode>SELECT<inlineCode> statement to retrieve the <inlineCode>ssn<inlineCode> column and the corresponding scrambled value using the <inlineCode>scramble<inlineCode> UDF. We can compare the original and scrambled values to ensure that the scrambling is working as expected.
Step 6: Monitor and maintain the scrambled data
To monitor and maintain the scrambled data, we can regularly check the sensitive columns to ensure that they are still rearranged and that there are no vulnerabilities or breaches. We should also maintain the scrambling key and UDF to ensure that they are up-to-date and effective.
Different Options for Scrambling Data in Redshift
Selecting a data scrambling technique involves balancing security levels, data sensitivity, and application requirements. Various general algorithms exist, each with unique pros and cons. To scramble data in Amazon Redshift, you can use the following Python code samples in conjunction with a library like psycopg2 to interact with your Redshift cluster. Before executing the code samples, you will need to install the psycopg2 library:
Random
Utilizing a random number generator, the Random option quickly secures data, although its susceptibility to reverse engineering limits its robustness for long-term protection.
Shuffle
The Shuffle option enhances security by rearranging data characters. However, it remains prone to brute-force attacks, despite being harder to reverse-engineer.
Reversible
By scrambling characters in a decryption key-reversible manner, the Reversible method poses a greater challenge to attackers but is still vulnerable to brute-force attacks. We’ll use the Caesar cipher as an example.
Custom
The Custom option enables users to create tailor-made algorithms to resist specific attack types, potentially offering superior security. However, the development and implementation of custom algorithms demand greater time and expertise.
Best Practices for Using Redshift Data Scrambling
There are several best practices that should be followed when using Redshift Data Scrambling to ensure maximum protection:
Use Unique Keys for Each Table
To ensure that the data is not compromised if one key is compromised, each table should have its own unique key pair. This can be achieved by creating a unique index on the table.
Encrypt Sensitive Data Fields
Sensitive data fields such as credit card numbers and social security numbers should be encrypted to provide an additional layer of security. You can encrypt data fields in Redshift using the ENCRYPT function. Here's an example of how to encrypt a credit card number field:
Use Strong Encryption Algorithms
Strong encryption algorithms such as AES-256 should be used to provide the strongest protection. Redshift supports AES-256 encryption for data at rest and in transit.
Control Access to Encryption Keys
Access to encryption keys should be restricted to authorized personnel to prevent unauthorized access to sensitive data. You can achieve this by setting up an AWS KMS (Key Management Service) to manage your encryption keys. Here's an example of how to restrict access to an encryption key using KMS in Python:
Regularly Rotate Encryption Keys
Regular rotation of encryption keys ensures that any compromised keys do not provide unauthorized access to sensitive data. You can schedule regular key rotation in AWS KMS by setting a key policy that specifies a rotation schedule. Here's an example of how to schedule annual key rotation in KMS using the AWS CLI:
Turn on logging
To track user access to sensitive data and identify any unwanted access, logging must be enabled. All SQL commands that are executed on your cluster are logged when you activate query logging in Amazon Redshift. This applies to queries that access sensitive data as well as data-scrambling operations. Afterwards, you may examine these logs to look for any strange access patterns or suspect activities.
You may use the following SQL statement to make query logging available in Amazon Redshift:
The stl query system table may be used to retrieve the logs once query logging has been enabled. For instance, the SQL query shown below will display all queries that reached a certain table:
Monitor Performance
Data scrambling is often a resource-intensive practice, so it’s good to monitor CPU usage, memory usage, and disk I/O to ensure your cluster isn’t being overloaded. In Redshift, you can use the <inlineCode>svl_query_summary<inlineCode> and <inlineCode>svl_query_report<inlineCode> system views to monitor query performance. You can also use Amazon CloudWatch to monitor metrics such as CPU usage and disk space.
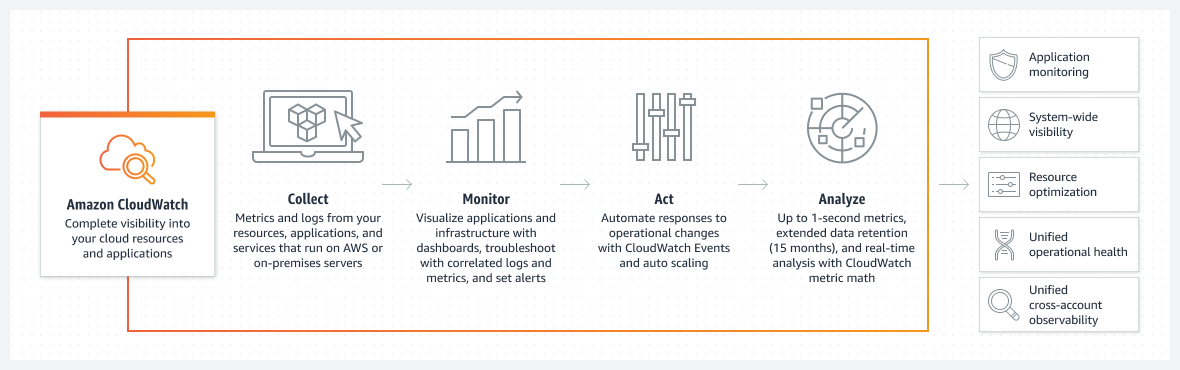
Establishing Backup and Disaster Recovery
In order to prevent data loss in the case of a disaster, backup and disaster recovery mechanisms should be put in place. Automated backups and manual snapshots are only two of the backup and recovery methods offered by Amazon Redshift. Automatic backups are taken once every eight hours by default.
Moreover, you may always manually take a snapshot of your cluster. In the case of a breakdown or disaster, your cluster may be restored using these backups and snapshots. Use this SQL query to manually take a snapshot of your cluster in Amazon Redshift:
To restore a snapshot, you can use the <inlineCode>RESTORE<inlineCode> command. For example:
Frequent Review and Updates
To ensure that data scrambling procedures remain effective and up-to-date with the latest security requirements, it is crucial to consistently review and update them. This process should include examining backup and recovery procedures, encryption techniques, and access controls.
In Amazon Redshift, you can assess access controls by inspecting all roles and their associated permissions in the <inlineCode>pg_roles<inlineCode> system catalog database. It is essential to confirm that only authorized individuals have access to sensitive information.
To analyze encryption techniques, use the <inlineCode>pg_catalog.pg_attribute<inlineCode> system catalog table, which allows you to inspect data types and encryption settings for each column in your tables. Ensure that sensitive data fields are protected with robust encryption methods, such as AES-256.
The AWS CLI commands <inlineCode>aws backup plan<inlineCode> and <inlineCode>aws backup vault<inlineCode> enable you to review your backup plans and vaults, as well as evaluate backup and recovery procedures. Make sure your backup and recovery procedures are properly configured and up-to-date.
Decrypting Data in Redshift
There are different options for decrypting data, depending on the encryption method used and the tools available; the decryption process is similar to of encryption, usually a custom UDF is used to decrypt the data, let’s look at one example of decrypting data scrambling with a substitution cipher.
Step 1: Create a UDF with decryption logic for substitution
Step 2: Move the data back after truncating and applying the decryption function
In this example, encrypted_column2 is the encrypted version of column2 in the temp_table. The decrypt_substitution function is applied to encrypted_column2, and the result is inserted into the decrypted_column2 in the original_table. Make sure to replace column1, column2, and column3 with the appropriate column names, and adjust the INSERT INTO statement accordingly if you have more or fewer columns in your table.
Conclusion
Redshift data scrambling is an effective tool for additional data protection and should be considered as part of an organization's overall data security strategy. In this blog post, we looked into the importance of data protection and how this can be integrated effectively into the data warehouse. Then, we covered the difference between data scrambling and data masking before diving into how one can set up Redshift data scrambling.
Once you begin to accustom to Redshift data scrambling, you can upgrade your security techniques with different techniques for scrambling data and best practices including encryption practices, logging, and performance monitoring. Organizations may improve their data security posture management (DSPM) and reduce the risk of possible breaches by adhering to these recommendations and using an efficient strategy.
<blogcta-big>